Abstract
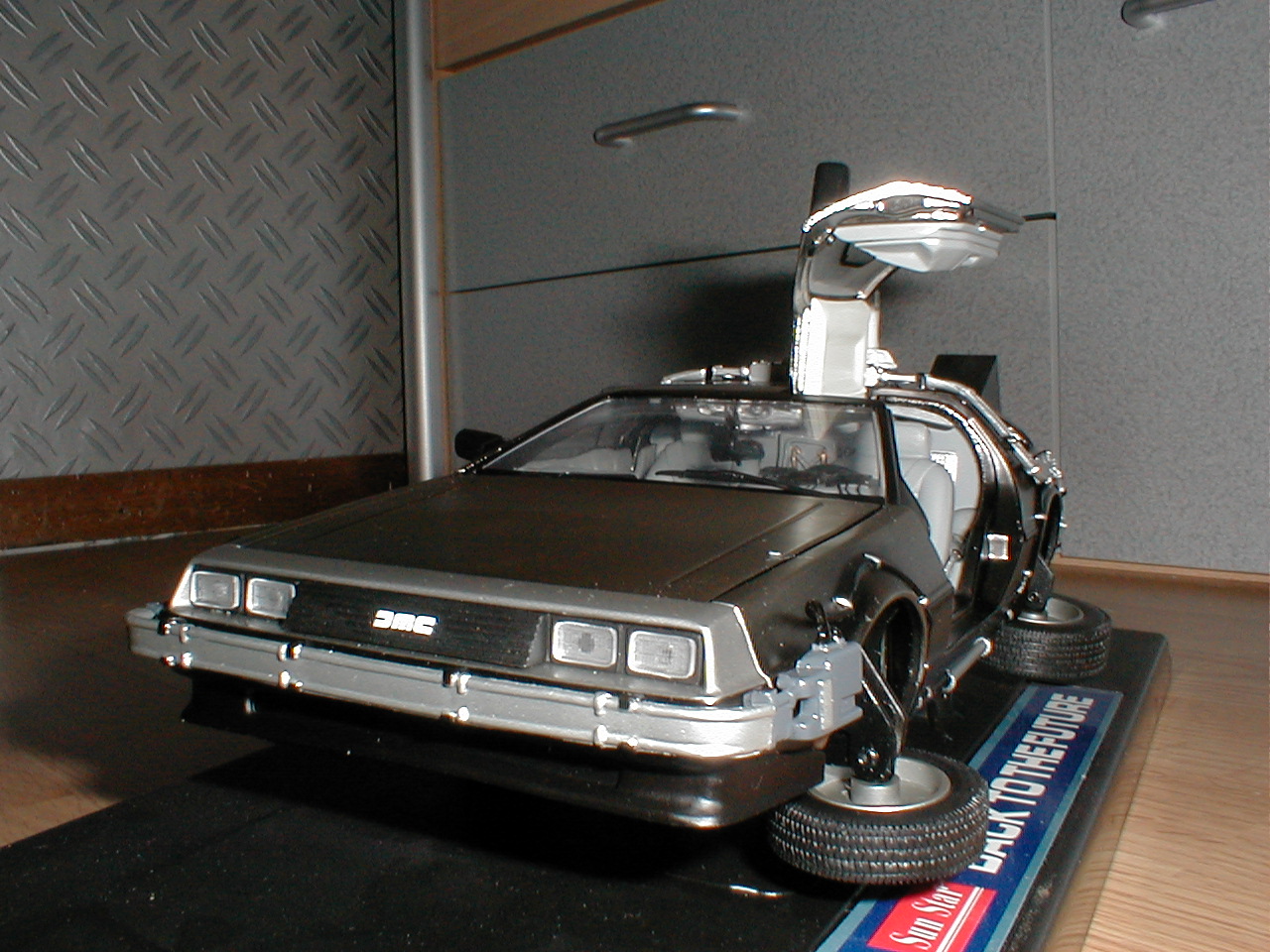
Introduction
The concept of Generative AI encompasses machine learning models capable of generating text, images, music, and more based on input data patterns. Unlike traditional AI, which primarily focuses on classification and analysis, Generative AI aims to create new content that mimics the characteristics of the training data. The emergence of advanced neural network architectures, particularly Generative Adversarial Networks (GANs) and Transformers, has propelled the capabilities of Generative AI, enabling innovative applications across various domains.
Mechanisms of Generative AI
One of the critical components driving Generative AI is the architecture of neural networks. Key frameworks include:
- Generative Adversarial Networks (GANs): Proposed by Ian Goodfellow in 2014, GANs consist of two neural networks— the generator and the discriminator. The generator creates new data instances while the discriminator evaluates them against real data. This adversarial process continues until the generator produces data indistinguishable from real samples.
- Transformers: Transformers, introduced by Vaswani et al. in 2017, have transformed the landscape of natural language processing (NLP). The self-attention mechanism in Transformers allows models to weigh the importance of different words relative to one another, significantly improving the quality of generated text. Notable examples include OpenAI's GPT-3 and later iterations, which leverage Transformers to generate human-like text.
- Variational Autoencoders (VAEs): VAEs are another popular generative model that incorporates variational inference. They work by encoding input data into a lower-dimensional space and then decoding it back to reconstruct the original data, allowing for the generation of new instances that share similar characteristics.
Recent Advances
Recent scholarly work and breakthroughs in Generative AI have influenced various sectors, leading to several notable developments:
- Text Generation: OpenAI's ChatGPT has gained widespread recognition for natural language generation, enabling users to create coherent and contextually relevant text. Further advancements include fine-tuning these models for specific tasks such as legal document drafting, technical writing, and creative storytelling, improving efficiency and quality.
- Image Synthesis: Models like DALL-E and Stable Diffusion have pushed the boundaries of image generation by using textual descriptions to create original artwork. The potential for personalized marketing campaigns and custom art production demonstrates the commercial viability and artistic potential of Generative AI in visual domains.
- Music Composition: AI-generated music has made significant inroads, with systems like OpenAI’s MuseNet generating complex compositions in various styles. These technologies not only assist composers but also democratize music creation by allowing individuals without formal training to generate high-quality compositions.
- Video Generation: The recent advancements in video synthesis enable Generative AI to create and manipulate video content. Research on models capable of generating short clips from textual descriptions promises to revolutionize the media and entertainment industry by lowering production costs and enhancing creativity.
- Multimodal Models: Models that can handle multiple forms of data input (text, audio, images) have seen significant progress. Examples include CLIP (Contrastive Language-Image Pre-training), which correlates images and text, enabling new applications in search and retrieval systems.
Applications of Generative AI
The potential impact of Generative AI spans a multitude of fields:
- Entertainment and Gaming: Generative AI facilitates the creation of immersive environments and non-player characters (NPCs) in video games, enhancing player engagement. Customizability allows players to generate their quests and adventures, tailoring experiences to their preferences.
- Healthcare: In the healthcare sector, Generative AI aids in creating synthetic medical data, which is crucial for research without compromising patient confidentiality. Additionally, it can assist in drug discovery by generating potential molecular structures.
- Marketing and Advertising: Generative AI streamlines the creation of personalized content for marketing campaigns. By analyzing consumer data, AI can craft tailored advertisements, leading to increased engagement and conversion rates.
- Fashion Design: Fashion brands are increasingly leveraging Generative AI to explore new design patterns and textures, enabling faster prototyping and reducing time-to-market for new collections.
- Education: AI-driven tools generate personalized learning content and simulations in educational settings, catering to diverse learning styles and improving overall engagement.
Challenges and Ethical Considerations
Despite its promising potential, Generative AI also poses several challenges and ethical dilemmas:
- Misinformation: The capacity to generate realistic text and media raises concerns regarding the proliferation of misinformation and deepfakes. It can contribute to social media manipulation and disinformation campaigns, threatening public trust.
- Intellectual Property: The legal implications surrounding the ownership of AI-generated content remain ambiguous. Questions arise regarding copyright and the rights of original creators versus AI's contributions.
- Bias and Fairness: Generative models often inherit biases present in their training data, resulting in generated content that may perpetuate stereotypes or reinforce societal biases. Addressing these biases is critical for creating fair and equitable AI systems.
- Environmental Impact: The computational resources required for training large models leave considerable carbon footprints. As the focus on sustainability grows, finding energy-efficient training methods is essential.
- Data Privacy: The extensive use of datasets for training models raises concerns about the privacy of individuals whose data might be included. Ensuring data anonymization and adherence to privacy laws is paramount.
Future Directions
As we look towards the future of Generative AI, the following trends and areas of research are anticipated:
- Improved Interaction with AI: Advancements in natural language understanding will lead to more intuitive interfaces, allowing users to interact seamlessly with Generative AI across platforms.
- Enhanced Customization: Future models may allow for personalized adjustments based on users’ preferences, leading to more refined and suitable content generation.
- Cross-Disciplinary Applications: The intersection of Generative AI with fields like neuroscience, psychology, and sociology will likely yield innovative solutions that address complex societal challenges.
- Robustness and Safety: Ongoing research into creating more robust models that can resist adversarial attacks and produce safe outputs is essential for widespread commercial adoption.
- Policy Development: Establishing guidelines and policies to govern the ethical and responsible use of Generative AI language model efficiency [Pin.it] will be critical in ensuring its benefits are maximized while minimizing risks.
Conclusion
Generative AI stands at the forefront of technological innovation, with the potential to reshape industries and enhance creativity. While its capabilities are remarkable, it is crucial to navigate ethical challenges and societal implications responsibly. Ongoing research, robust policy frameworks, and interdisciplinary collaboration will be vital in realizing the full promise of Generative AI while mitigating its risks. As we move forward, the synergy between human ingenuity and Generative AI could lead to unprecedented advancements, marking a new era in technology.
References
- Goodfellow, I., et al. (2014). "Generative Adversarial Nets." Advances in Neural Information Processing Systems.
- Vaswani, A., et al. (2017). "Attention Is All You Need." Advances in Neural Information Processing Systems.
- Radford, A., et al. (2021). "Learning Transferable Visual Models From Natural Language Supervision." Proceedings of the International Conference on Machine Learning.
- OpenAI. (2023). "GPT-4: Technical Report."
- Dhariwal, P., & Nichol, A. (2021). "Diffusion Models Beat GANs on Image Synthesis." arXiv preprint arXiv:2105.05233.
This comprehensive examination illustrates not only the capabilities of Generative AI but also highlights the challenges and ethical considerations inherent in its application. The journey of Generative AI is just beginning, and its influence will undoubtedly expand in the years to come.